In recent years, disruptive and innovative technologies — namely artificial intelligence (AI), cloud computing and edge computing — in the video analytics arena has resulted in increasing demand for security and non-security applications.
Cloud-based video analytics help reduce overall costs as storage space and computing efforts are distributed over the cloud server. Edge-based video analytics help to process the data on-premises, thereby reducing bandwidth and cloud loads while accelerating the analysis process. With vendors and systems integrators helping end customers achieve newfound security and business intelligence capabilities, the overall marketplace is poised for significant growth. For instance, according to a report by MarketsandMarkets Research, the global video analytics market — estimated to be worth $7.1 billion in 2022 — is projected to increase to $20.3 billion by 2027. That amounts to a compound annual growth rate (CAGR) of 23.4 percent.
Ahead, security professionals who specialize in the space detail the hottest market niches for AI-enabled video analytics, best practices for overcoming deployment challenges, and how integrators can justify the cost of intelligent analytics with their end customers. You’ll also learn how intelligent analytics is helping mitigate false positives.
Top Use Cases
Once viewed as a security luxury that came at a premium — even though limited in form and application — today’s video analytics that harness AI and its subsets are becoming ever more commonplace across a widening array of end-user markets.
Quang Trinh, manager, professional services for Axis Communications, Chelmsford, Mass., points to human and vehicle tailgating scenarios, perimeter protection, crowd monitoring and behavioral insight as just some of the top use cases nowadays for AI-based analytics.
Human and vehicle tailgating solves a lot of access control pain points as far as unauthorized access control, Trinh explains. Many tailgating vulnerabilities are tied to user behavior. AI can better detect and classify these vulnerabilities in real time and capture historical data, which can provide the end customer with a path to correct internal and external trends by providing actionable information to move toward a more proactive approach to physical access control. Notifications in real time — when an AI analytics detects tailgating combined with a human verification of the incident — will drive down false positives and provide a more efficient response.
“AI-based perimeter protection use cases involve a higher level of detection and classification of human and vehicle threats,” Trinh says. “Other anomalies that would cause false positives in this use case are eliminated through AI’s deep learning models’ ability to detect only human and vehicle-based threats. This applies to images, videos, thermal imagery and radar data that benefit from a deep learning model on human and vehicle detection. With advancements in classifying objects, threats that move beyond humans and vehicles can be detected and classified, such as bags, pieces of luggage and packages left behind.”
Crowd monitoring scenarios such as occupancy data are more accurate with AI-based analytics, where human classification performs better than pixel-based video analytics, Trinh explains. In real-world environments, factors such as lighting, shadows and human density can reduce the accuracy of the count data. When multiple cameras that can detect and classify humans based on AI are used for occupancy in a scene, the accuracy increases and the additional metadata provided can also be used to measure time-in-area along with count data.
“Trajectory data can also be captured, and historical trends from that data can account for which direction has the most traffic versus lighter traffic,” Trinh adds. “Along with occupancy data, IP cameras provide video data that correlates with the analytics data, which can be very useful in the end customer understanding trends and statistics about their business through occupancy — an added benefit of the camera being used for both physical security and operational efficiencies.”
Restaurants, schools, hotels, retailers, cannabis dispensaries, healthcare facilities, smart cities and more use analytics to count how many people go in and out of buildings and at what time to understand when lines are long, or identify where in a building customers are spending their time, explains Ken Francis, president, Eagle Eye Networks, Austin, Texas.
“Where AI-enhanced analytics is really making a difference is in accuracy and ease of deployment,” Francis says. “By leveraging AI analytics in the cloud, you can get highly accurate information, and it’s very simple for integrators to deploy. Analytics in the cloud literally can be turned off or on with a simple flip of the switch. Businesses can get valuable video analytics data from existing cameras by leveraging cloud video surveillance. There’s no need to stand up extra onsite peripheral equipment.”
Proactive video monitoring is one area, Francis cites, where Eagle Eye Networks is seeing tremendous benefit from AI-filtered analytics. When combining cloud-based video, AI analytics, and live-audio voice downs with proactive video monitoring, a crime can be stopped before it happens instead of just recording it, he explains.
“The AI distinguishes between actionable and non-actionable events, so security personnel can focus on important events,” he says. “Security will then proactively use audio equipment to voice down to let a suspicious person know that they are trespassing and they should leave the premises immediately. This type of proactive video monitoring is successful in deterring criminal incidents more than 90 percent of the time.”
AI-enhanced analytics were historically used to enable alerts using rules such as line crossing or loitering. Today, in addition to rule-based alerts, the detection and classification capabilities of AI-enhanced analytics make searching videos more efficient. // IMAGE COURTESY OF MOTOROLA SOLUTIONS
Michael Wilson, vice president of integrated solutions for Pavion, Chantilly, Va., also emphasizes the growing opportunities around proactive video monitoring, and especially when coupled with talk-down functionality.
“We are leveraging analytics at the edge to do the people detection, and then it triggers alert notifications to our central monitoring station. Our agents are then in real time able to remote in and do live talk down through the IP-based loudspeaker that would be on site,” Wilson explains.
Loitering is a huge issue that integrators such as Pavion are helping end customers combat by leveraging AI-enhanced analytics. Here as well proactive video monitoring is proving highly useful.
“In real time we can use the analytics of a camera or the NVR to detect objects, such as people entering virtual fence lines, and then react to what those people are doing,” Wilson says. “In the case of somebody who is just walking down the street, we’re going to detect them coming into frame and everything’s fine. But if we have triggers that are set for loitering — if a person stays in frame for a prolonged period of time — the video analytics will trigger notifications back to an active monitoring station to investigate and to look at what’s going on in that moment.”
Behavioral insight applications that leverage AI-based audio analytics are highly beneficial to protect people and provide awareness of real-time threats, Trinh says, such as detecting aggression and elevated noise including screaming and even gunshots.
“The fusion of audio and video data provides very efficient analytics by adding context to a situation,” he explains. “Many IP cameras have audio capabilities or can pair with audio devices that reside on the network. AI-based audio analytics process the auditory data in real time and have proven valuable in scenarios where aggression detection paired with video provided proactive information for end customers to react to. From enterprise businesses to schools, retail and hospitals, these customers are utilizing audio analytics with video to provide a more efficient process to maximize their current system.”
Louis Rabenold, director of VMS products for Vicon, Hauppauge, N.Y., says security integrators are embracing AI-enhanced analytics and discovering innovative ways of pairing traditional analytics (e.g., motion detection, intrusion and line cross) with object-based classification (e.g., the ability to determine a person or vehicle within a scene).
By combining these two unique categories of analytics, integrators are using them to address longstanding issues in pioneering ways, Rabenold says. For example, one of Vicon’s integrators was tasked with securing a long-term parking lot that was experiencing ongoing incidents of catalytic converter thefts.
“Deploying a motion-based analytic resulted in too many false positives. Instead, they implemented a loitering alarm paired with a ‘people’ object classification that would trigger only when the camera detected a person remaining in an area for too long,” he describes. “The result? They were alerted whenever a person lingered in the same spot for five minutes, but they weren’t alerted by the numerous occurrences of vehicles [stationary and moving] or people walking.”
Mike Reading, product engineering team lead at i-PRO Americas, Houston, says one of the biggest areas of deployment the company has seen is in the K-12 market where edge-based analytics offer an affordable way for schools with limited resources to expand coverage in areas that would be impossible to cover with existing staff. With AI-based object detection, it’s easy to set up rules to send alerts when students are in places they should not be, especially after hours.
“Loitering of people or vehicles is nearly impossible to detect without AI-assisted algorithms, yet it’s a critical part of a proactive security stance,” Reading says. “Most importantly, AI-enhanced analytics are enabling our users to react to events in real time as opposed to only reacting to events in the past. By making AI standard on most of our camera lines, we’re helping to democratize AI in video security so that it’s an available part of the toolset that can be deployed at any time.”
Overcoming Deployment Challenges
Among the challenges integrators often face when deploying AI and analytics on customer systems is the initial configuration and setup and how long that can take, especially on large systems with many cameras. Analytics rules, for example, such as detecting the presence of an object in an area of the view, typically have to be configured on each camera.
“Some of the most recent AI technologies Motorola Solutions has created overcome this obstacle by operating right out of the box with no configuration required,” says Kaveh Malakuti, director of product management, Motorola Solutions’ Vancouver, B.C., Canada-based Avigilon brand. “An example is our Unusual Activity Detection (UAD) technology, which automatically learns what is normal in a scene in terms of the speed at which people or vehicles are moving or the location within the scene, and can then automatically inform security operators of anomalous events through Avigilon Control Center’s Focus of Attention display.”
Cost is still a barrier to adoption, so building a business case around specific pain points to demonstrate return on investment (ROI) will be time well spent, advises Jason Burrows, sales director, IDIS America, Coppell, Texas. Proof of concepts using a handful of edge AI cameras or a simple add-on AI box device to tackle crime, theft, fraud, common accidents or loss prevention challenges will quickly demonstrate that a proactive approach to security and safety will positively impact the bottom line and boost profitability.
“Implementing AI video no longer needs to be complex or expensive,” Burrows says. “Integrators can also look toward vendors that have eliminated complicated boundary recognition area and rule configuration associated with AI cameras to make them faster and more affordable to install, and in turn, a more attractive proposition for small to medium-sized businesses.”
There are a raft of different options now available that won’t increase storage costs, such as easily installed add-on devices that come with zero calibration and efficient analytics, Burrows adds. “This enables integrators to offer a compelling AI solution that eliminates complicated software, upfront license and maintenance fees, ongoing device connection costs, or the need for customers to replace existing cameras with edge AI models or deploy expensive AI NVRs.”
Matt Mulham, marketing manager, Speco Technologies, Amityville, N.Y., says the biggest barrier to begin deploying AI-enabled analytics is frankly lack of education. It does not help that end customers oftentimes carry a predisposition toward advanced analytics.
“The customers believe that analytics are too ‘high-tech’ or simply don’t understand all of the possible use cases for analytics,” he says. “That is why we strive to educate all of our integration partners and end-user customers alike on the ease of Speco Technologies’ advanced analytics. We house numerous ‘Quick Hits’ or video tutorials on both our website and YouTube page outlining each analytic step by step in an easy-to-understand manner.”
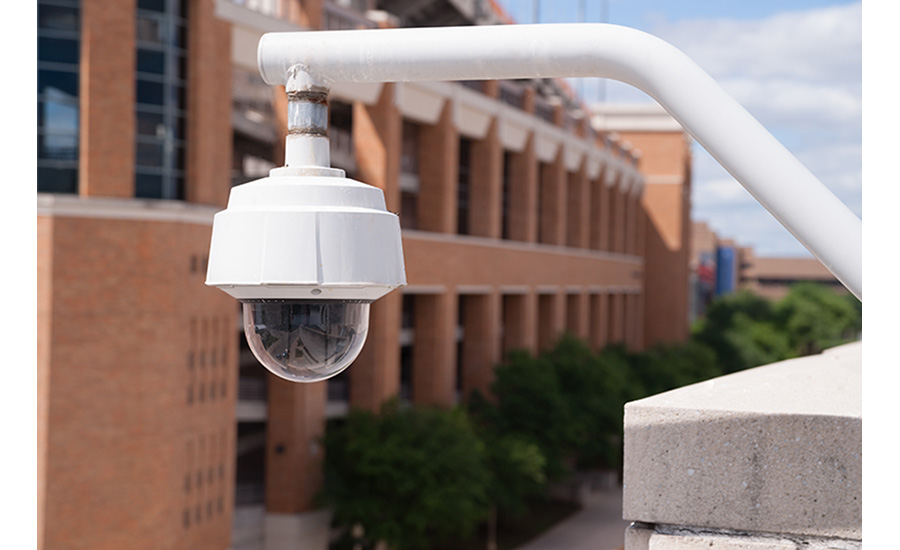
Edge-based analytics deployed in the K-12 market offer an affordable way for schools with limited resources to expand coverage in areas that would be impossible to cover with existing staff. // CHRISBOSWELL/ISTOCK / GETTY IMAGES PLUS VIA GETTY IMAGES
Continuous engagement with the customer is needed to maximize the system’s performance once AI-based analytics have been integrated into existing systems and processes, Trinh says.
“Many people view AI-based analytics from vendors as a magical black box that will solve their customer’s needs, and this is the first misconception of AI,” Trinh says. “AI, deep learning and machine learning are tools that will greatly improve, automate and make the current system more efficient. Integrators are already designing systems for their customers, and AI-based analytics is just one piece of the design. Integrators who understand how powerful this tool can be will apply the right analytics in the solution they design for their customers. AI-based analytics span cloud, on-premise and edge device solutions and are more of an ecosystem solution that involves more than one vendor.”
The factors that have long impacted video in the security industry also apply to AI-based analytics and its deployment, he adds. Weather, lighting, image quality and quality hardware will have major implications on the performance of the analytics.
“GIGO — or garbage in, garbage out — is a term used in deep learning and machine learning to ensure that the quantity and quality of data are equally important and provide a more accurate outcome,” Trinh says.
Setting proper expectations with customers is paramount, says Rabenold, who views integrators as instrumental in helping end users properly identify issues that can be addressed with AI — and which can’t. “They’re on the front lines of ensuring the installation and camera set-up is proper for the optimal AI experience,” he adds. “And they’ll need to help the customer understand that they may need to engage with the system in the beginning to ensure they are getting the most from the AI with the proper rules, events, alarms, searches and notifications that will fit their needs.”
Deploying video analytics traditionally requires integrators to make complex calibrations, Francis says, and ensuring the camera’s environment is as controlled as possible. This is expensive and time consuming and if not done correctly, either the analytics would not work, or worst case, they would give incorrect information. “Deploying cloud-based AI analytics instead of pixel-based analytics takes away this challenge,” he says. “There is no need for manual calibration, the installation is straightforward, and the software is maintained and updated by the cloud provider.”
One of the biggest challenges is that not all devices or analytics are “set and forget,” says Brad McMullen, president of 3xLOGIC, Fishers, Ind. Oftentimes, the camera may need to be adjusted after a few days or weeks of data is gathered. “During this time period, the analytic may not provide the exact data the customer is looking for so setting expectations up front about the process is critical,” he adds.
A top challenge that Reading observes integrators contend with is ensuring a proper viewshed for the camera. AI processes are very specific in what they are designed to identify, so ensuring that the view of the camera is within tolerance for the specific AI application limits, such as minimal pixel density at a given distance, can ensure optimum results. These challenges can be overcome by leveraging the wide variety of tools, he says.
“i-PRO offers a system design tool that can be used to ensure that the correct camera is being used, along with the i-PRO configuration tool which is used after the camera is installed to verify that it is providing an image that meets the specifications for the AI application in use,” Reading says. “Of course, the best AI-based camera is of no use if it’s not paired with a compatible VMS that can read all the advanced metadata being generated by the camera. It’s imperative that the VMS and any cameras be fully compatible, beyond simple ONVIF support, to make full use of AI-based analytics.”
With the increased accuracy of video analytics systems, Florian Matusek, director of video analytics for Montreal-based Genetec, stresses that scalability can present considerable technical challenges for integrators and end users. So, then, how can a video analytics system be deployed not only on a few cameras but on a large quantity or potentially all cameras on a site?
To begin drilling down into that multifaceted answer, Matusek, who also serves as managing director of Genetec’s Vienna office, says integrators should ask the following questions: “Does the system scale automatically? Does it support load balancing across servers? Does it allow exporting and importing configurations of cameras to deploy them on other similar views? Are extensive training, certification and professional services available in my region?”
Joshua Akre, performance engineering manager at Milpitas, Calif.-based systems integrator Northland Controls, echoes similar challenges with largescale or enterprise-level deployments of video analytics.
“There are end users large enough to the point that they can’t effectively deploy analytics without a very sizable project and without incredibly thorough vetting,” he explains. “So they are taking a little bit longer to adopt some of these platforms across their entire camera environment. But that’s where they have to be smart about it; for example, going after perimeter doors or going after high value rooms where there can be almost an immediate impact.”
Thoroughly scoping out the project and establishing standards for the deployment are key to heading off and minimizing technical difficulties, Akre says. So are customer expectations and knowledge about the solution being deployed.
“There are camera systems that come with analytics readily available. But if you don’t have time allocated to set up the analytics to adjust and calibrate them as needed, then you may just end up with a really powerful camera taking video at a door or a space. So the analytics may not be utilized if the customer doesn’t know about them or if it just isn’t an expectation,” Akre explains.
Such a scenario can result in many underused cameras and analytics, which can pose a wholly different headache upon returning to the site post deployment or when the customer ends up playing around with some of their cameras and discovers new feature sets.
“Then you have to have that secondary conversation about what exactly they are looking to gain out of those. And sometimes it can be a really quick calibration or even just setting up trip lines within the camera,” Akre says. “It comes down to initially scoping out what the customer needs that video system for.”
Automated license plate recognition, in tandem with AI-enabled video analytics, can provide an affordable, practical solution designed for businesses seeking maximum flexibility and scalability. // IMAGE COURTESY OF EAGLE EYE NETWORKS
Justifying the Cost of Intelligent Analytics
Justifying the cost of a deployment with the end customer ultimately will come down to ROI, says Trinh of Axis Communications. Consider: Intelligent video analytics can reduce risk, liability and improve operational efficiencies that can be monetized.
Reducing risk is accomplished when statistical and historical data from intelligent video can prepare an organization to identify current and future risk trends in their environment. Images and videos can provide a lot of contextual and spatial information.
“Many customers are already mitigating costly liabilities with intelligent video data,” Trinh says. “AI-based analytics is a tool that can reduce those liability costs. For example, a retailer or business that consistently gets fined for having their emergency exits blocked can see costs varying from three to six figures based on the number of offenses. The cost to deploy an AI-based analytic will warrant the reduction in fines that occurred over a period of time.”
The reduction in liability is also lessened if the customer is ensured that the emergency exits are not blocked. In a real-world situation, when customers or people need to vacate a business through an emergency exit, having it blocked can also lead to a legal risk that the organization would like to avoid.
Trinh explains that operational efficiencies can be improved through automation and by reducing long, repetitive human tasks with AI-based solutions. A human-centric approach is still required to validate and make final decisions from the system. Many organizations are approaching a methodology around doing more with less, he says.
“Many organizations already have video systems in place for public safety, so many are asking what else these systems can provide,” Trinh adds. “Deep learning really thrives on processing images and videos. Many organizations are looking to this deep learning analytics to provide more insight from the already valuable video data that is being created.”
McMullen of 3xLOGIC strongly advises integrators to identify all the different use cases for the end customer. Avoid getting tunnel vision about a security solution and be sure to consider health and safety applications, business intelligence opportunities and compliance assurance. “Ensure the customer has the opportunity to track where the advanced analytics are being used and what benefits are being provided to substantiate ROI and identify opportunities for expanded applications,” he says.
Video security systems have evolved far beyond the obligatory “sunk cost” expense of the past, says Reading of i-PRO. With intelligent video analytics, organizations can proactively protect people and assets with less resources, all the while enabling security and operations teams to be more proactive in their response to events, rather than simply reacting to events that have happened. Businesses can also use their AI-based video surveillance systems to provide a wealth of valuable data about operations efficiency and even map customer behavior in stores, Reading explains.
“Used correctly, this new breed of camera becomes a data gathering tool that can monitor room occupancy, read license plates, measure sales conversion rates, notify when stock is running low and much more,” he says. “Each management team will likely envision different opportunities once they understand what is possible. With the capacity to enhance revenue generation and support operational efficiency, it’s possible for such systems paying for themselves.”
Matusek of Genetec advises integrators succinctly: Start with the problem, not with the solution. He says to first make a list of all problems that need to be solved in your area of responsibility. Next, select the ones where video analytics could be a solution. And finally, check each of them for viability and if video analytics is the best tool for the job. “Once you’ve drilled down and identified the actual business problem that you would like to solve, it’s usually easy to build a business case around it,” he says. “The most important thing is to solve a real problem and not to deploy video analytics for the sake of it.”
Analytics add considerable value for real-time workflows and forensic, post-incident investigations, says Malakuti of Motorola Solutions. The most obvious is the time saved by using AI, which translates to financial benefits. For example, in the past, investigations to find a specific person could take days of reviewing recorded video. Today, it can be done in a matter of minutes or seconds with AI saving customers enough to cover their costs quickly, he says.
“The second is reducing fatigue and stress for operators by using a more efficient system,” Malakuti adds. “Analytics allow customers to create repeatable human curated workflows that save training time and deliver more consistent performance with infinite staying power.”
Francis of Eagle Eye Networks says integrators will not have to spend a lot of time justifying the cost of intelligent video analytics in a cloud-based system because it’s cost effective, and easy to operate.
“The C-suite is understandably reluctant to make risky investments in expensive specialty cameras, standing up servers, and paying for ongoing maintenance just to try out video analytics,” he says. “However, that risk is removed when your AI and analytics are in the cloud. You can try out video analytics anytime you want and turn them off if they’re not serving your business needs.”
Features such as video analytics can be added — to one, several or all cameras — at any time. The analytics are delivered via the cloud when a business owner wants them. They can literally turn them on for a month, try them out and turn them off. They pay by subscription for what they’re using.
“Some cloud VMS providers even offer certain video analytics — such as new AI-powered video search — as a no-extra-cost standard feature of cloud VMS,” Francis adds.
Akre of Northland Controls advises his fellow integrators it can be “incredibly strategic” to bring your manufacturer partners into conversations with the end customer. “I don’t think we’ve had a situation where we’ve deployed analytics and have not had the manufacturer in discussions with the customer. Be it the customer who is approving the budget or their IT or InfoSec [department] because it does become a little bit more of a partnership at that point,” he explains.
As the integrator, you’re not necessarily selling the customer. More so, you are facilitating that transaction, Akre adds. “[The manufacturer] has canned marketing material. They have sales engineers that can definitely help you out. And especially if this is new technology to your company, you don’t want to go in front of a customer and get caught in a situation where they expect you to know X,Y and Z.”